Topic Statistics how to find critical value: When working with statistics, knowing how to find the critical value is essential for accurate analysis. The critical value helps statisticians calculate the margin of error within a dataset, allowing them to draw meaningful conclusions and make informed decisions. By understanding the significance level of a hypothesis test, one can determine the critical value needed for accurate statistical analysis. This knowledge empowers researchers and analysts, enabling them to confidently interpret data and glean valuable insights.
Table of Content
- How to find the critical value in statistics?
- What is a critical value in statistics and why is it important?
- How is a critical value used to calculate the margin of error in a dataset?
- YOUTUBE: Finding critical values for hypothesis testing using z or t table
- What factors determine the significance level when finding a critical value?
- Can you explain the concept of upper-tailed and lower-tailed critical values?
- What are the steps involved in finding the critical value for a hypothesis test?
- Are there different methods or formulas to find the critical value depending on the type of statistical test? If so, what are they?
- How does the sample size affect the critical value and the overall statistical analysis?
- Are there any common distributions used to find critical values in statistics? If yes, which ones and how are they used?
- Can you provide an example or scenario where finding the critical value in statistics is crucial for accurate data analysis?
How to find the critical value in statistics?
To find the critical value in statistics, you need to follow these steps:
Step 1: Determine the significance level (α) of your hypothesis test. This is the level of confidence you want to have in your statistical inference. Commonly used values for α are 0.05 (95% confidence) and 0.01 (99% confidence).
Step 2: Determine if your hypothesis test is one-tailed or two-tailed. A one-tailed hypothesis test involves testing a specific direction (either a greater than or less than relationship), while a two-tailed test involves testing for any difference.
Step 3: Look up the critical value from a standard statistical table or use a calculator or software that provides critical values. The table or tool will provide critical values based on the significance level and the degrees of freedom associated with your test.
For example, if you are conducting a one-tailed hypothesis test with a significance level of 0.05 and have 20 degrees of freedom, you can use a t-distribution table to find the critical value. In this case, if you are looking for a critical value in the upper tail, you would look for the value closest to 0.05 in the table for 20 degrees of freedom. Let\'s say you find the critical value as 1.725.
Step 4: Calculate the test statistic for your sample data based on the specific hypothesis test you are conducting. This may involve performing calculations or using pre-defined formulas depending on the statistical test you are performing.
Step 5: Compare the test statistic to the critical value. If the test statistic exceeds the critical value, you can reject the null hypothesis. If the test statistic falls below the critical value, you fail to reject the null hypothesis.
Remember that finding the critical value is essential in hypothesis testing as it helps determine the boundary for accepting or rejecting the null hypothesis in favor of an alternative hypothesis.
READ MORE:
What is a critical value in statistics and why is it important?
In statistics, a critical value is a specific point on a sampling distribution that is used to determine the significance of a statistical test. It is primarily used in hypothesis testing, where it helps determine whether to reject or fail to reject a null hypothesis.
A critical value is important because it allows us to make decisions about the null hypothesis based on the observed data. It provides a threshold or cutoff point beyond which we consider the results to be statistically significant.
The critical value is determined by the significance level (also known as alpha level) of the test. The significance level is the probability of incorrectly rejecting the null hypothesis when it is actually true. It is typically set before conducting the test, depending on the desired level of confidence.
To find the critical value, you first determine the significance level for your hypothesis test. This can be denoted as α (alpha). The significance level is typically expressed as a decimal or percentage, such as 0.05 or 5%.
Once you know the significance level, you can determine the critical value using a statistical table or a statistical calculator. The critical value corresponds to the desired level of significance and the specific test being conducted (such as a one-tailed or two-tailed test).
For example, if you are conducting a two-tailed hypothesis test with a significance level of 0.05, you would need to find the critical value associated with a 0.025 significance level in each tail of the distribution. This critical value would determine the cutoff point for rejecting the null hypothesis in either direction.
By comparing the test statistic (calculated from the sample data) to the critical value, you can determine whether the test statistic falls in the critical region (reject the null hypothesis) or outside the critical region (fail to reject the null hypothesis). If the test statistic is greater than or less than the critical value, it provides evidence to suggest that the observed results are statistically significant.
In summary, a critical value in statistics is a point on a sampling distribution that helps determine the significance of a statistical test. It is important because it allows us to make informed decisions about the null hypothesis based on the observed data and desired level of confidence.
How is a critical value used to calculate the margin of error in a dataset?
To calculate the margin of error in a dataset using a critical value, follow these steps:
Step 1: Determine the significance level (α) for your hypothesis test. The significance level is typically given in decimal form, such as 0.05 or 0.01. It represents the maximum allowable probability of making a Type I error.
Step 2: Identify whether you have a one-tailed or two-tailed test. In a one-tailed test, the critical value is used to test for a difference in one specific direction (e.g., greater than or less than). In a two-tailed test, the critical value is used to test for a difference in either direction.
Step 3: Look up the critical value associated with the significance level and the type of test in a statistical table. For example, for a one-tailed test with a significance level of 0.05, you would look up the critical value corresponding to an area of 0.05 in the appropriate table. This critical value represents the cutoff point beyond which the test statistic would be considered statistically significant.
Step 4: Calculate the standard error of the statistic you are interested in. The standard error measures the average deviation of sample statistics from the population parameter.
Step 5: Multiply the standard error by the critical value obtained in Step 3. This product represents the margin of error.
Step 6: Interpret the margin of error. It provides an estimate of the maximum likely deviation of the sample statistic from the true population parameter. The margin of error is typically added and subtracted to the sample statistic to create an interval estimate.
By using the critical value associated with the desired significance level and test type, you can determine the margin of error in a dataset. Keep in mind that this method assumes the dataset follows an appropriate probability distribution and that the necessary assumptions for hypothesis testing are satisfied.
Finding critical values for hypothesis testing using z or t table
Are you struggling to understand critical values in statistics? Fear not, because in this video, we break down the concept into simple terms and provide clear examples to help you grasp this crucial statistical tool. Don\'t miss out on unlocking the power of critical values - hit play now!
What factors determine the significance level when finding a critical value?
The significance level, also known as the alpha level, is determined by the researcher or analyst conducting the statistical analysis. It represents the probability of making a Type I error, which is rejecting the null hypothesis when it is actually true.
To find the critical value, you need to consider the significance level chosen for the hypothesis test. The significance level is typically denoted as α (alpha) and is usually set at 0.05 or 0.01, but it can vary depending on the specific research or analytical requirements.
The significance level determines the critical value by controlling the likelihood of making a Type I error. A lower significance level (e.g., 0.01) means that the researcher wants stronger evidence before rejecting the null hypothesis. This leads to a smaller critical value, as the researcher is less willing to reject the null hypothesis based on a small amount of evidence.
Conversely, a higher significance level (e.g., 0.05) indicates a higher tolerance for Type I errors. In this case, a larger critical value is used, meaning the researcher is more willing to reject the null hypothesis based on weaker evidence.
So, the factors that determine the significance level when finding a critical value are the researcher\'s desired level of confidence in the results and the acceptable risk of making a Type I error. The specific significance level chosen depends on the nature of the study, the potential consequences of a Type I error, and other contextual factors.
Can you explain the concept of upper-tailed and lower-tailed critical values?
Sure! In statistics, the concept of upper-tailed and lower-tailed critical values is related to the type of hypothesis test being conducted.
When conducting a hypothesis test, statisticians often have two competing hypotheses: the null hypothesis (H0) and the alternative hypothesis (Ha). The null hypothesis represents the assumption or claim that is initially assumed to be true, while the alternative hypothesis represents the alternative claim that is being tested.
The critical value is a specific value that is compared to the test statistic (calculated from the data) to determine if there is enough evidence to reject the null hypothesis in favor of the alternative hypothesis. It helps in determining the critical region or the range of values of the test statistic that would lead to rejecting the null hypothesis.
Now, here\'s the difference between upper-tailed and lower-tailed critical values:
1. Upper-tailed critical value: This is relevant when the alternative hypothesis is looking for evidence of a positive effect or an increase in a parameter. In other words, it is used when the alternative hypothesis suggests that the population parameter is greater than a specific value. The upper-tailed critical value is the value beyond which the test statistic needs to fall in order for us to reject the null hypothesis. It represents the critical region in the upper tail of the distribution.
2. Lower-tailed critical value: This is relevant when the alternative hypothesis is looking for evidence of a negative effect or a decrease in a parameter. In other words, it is used when the alternative hypothesis suggests that the population parameter is smaller than a specific value. The lower-tailed critical value represents the value below which the test statistic needs to fall in order for us to reject the null hypothesis. It represents the critical region in the lower tail of the distribution.
The choice between upper-tailed and lower-tailed critical values depends on the specific research question and the alternative hypothesis being tested. It is important to correctly identify the appropriate critical value to use in order to make accurate conclusions about the hypothesis test results.
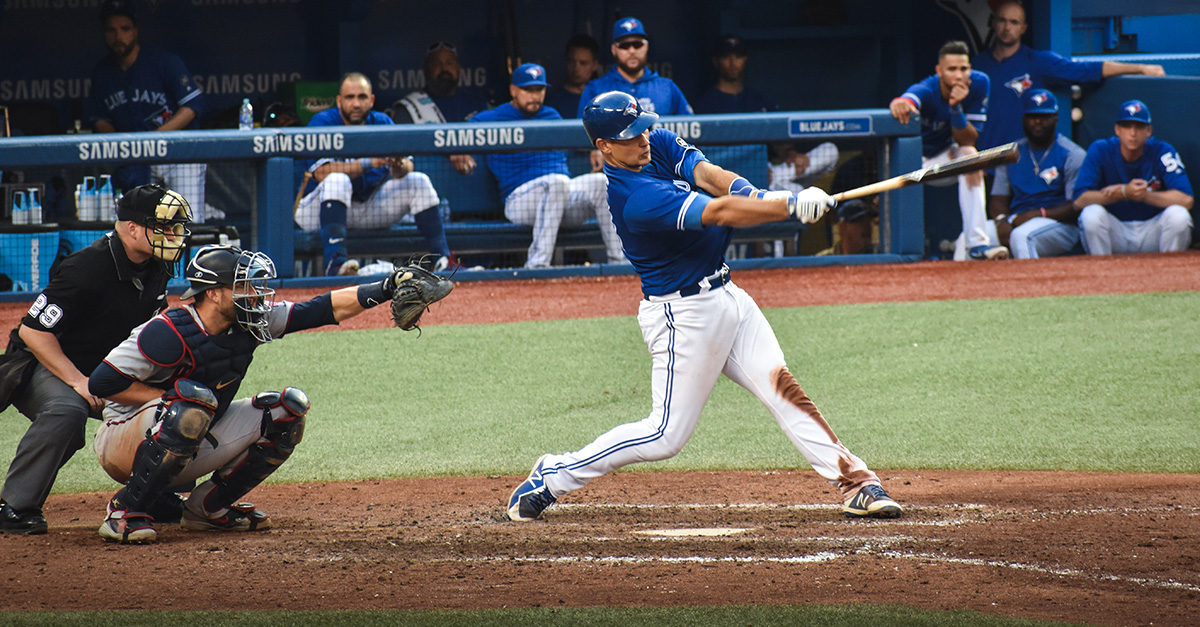
_HOOK_
What are the steps involved in finding the critical value for a hypothesis test?
To find the critical value for a hypothesis test, follow these steps:
1. Determine the significance level (α): The significance level, denoted by α, is the probability of observing a test statistic as extreme as the one calculated, assuming the null hypothesis is true. Common significance levels are 0.05 (5%) and 0.01 (1%), but they can vary depending on the specific test and the context.
2. Identify the test statistic distribution: The choice of test statistic distribution depends on the type of data and the nature of the hypothesis test. Common distributions include the standard normal distribution (Z-distribution) for large sample sizes and the t-distribution for small sample sizes.
3. Determine the tail(s) of the test: Depending on the alternative hypothesis, the test can be one-tailed or two-tailed. In a one-tailed test, the critical value is located in one tail of the distribution, either the left or right. In a two-tailed test, the critical value is split between both tails.
4. Look up the critical value in a statistical table: Statistical tables provide critical values corresponding to specific significance levels and degrees of freedom. Find the appropriate table for your chosen test statistic distribution and locate the row or column that corresponds to the desired significance level.
5. Consider the direction of the test: If the hypothesis test is an upper-tailed test (alternative hypothesis indicates a greater than relationship), the critical value will be found on the positive side of the distribution. If it is a lower-tailed test (alternative hypothesis indicates a smaller than relationship), the critical value will be found on the negative side. For a two-tailed test (alternative hypothesis indicates a not equal to relationship), the critical value will be split between the positive and negative sides.
6. Calculate the critical value: Once you have identified the row or column in the statistical table, locate the specific value that corresponds to the chosen significance level and direction of the test. This value represents the critical value for the hypothesis test.
Remember that the critical value divides the distribution into the rejection region and the non-rejection region. If the test statistic falls within the rejection region (beyond the critical value), you reject the null hypothesis; otherwise, you fail to reject the null hypothesis.
Finding critical values for confidence levels
Boost your statistical knowledge by diving into confidence levels with this informative video. Whether you\'re a student or a professional, understanding confidence levels is essential in drawing accurate conclusions from data. Join us as we walk you through step by step, demystifying this important statistical concept. Get ready to boost your confidence - click play to begin!
Are there different methods or formulas to find the critical value depending on the type of statistical test? If so, what are they?
Yes, there are different methods or formulas to find the critical value depending on the type of statistical test. The two commonly used methods to find critical values are:
1. Z-table: The Z-table is used to find critical values for tests involving the standard normal distribution (also known as the Z-distribution). In this method, you determine the critical value by finding the Z-score corresponding to a given significance level (α) or confidence level. The Z-score represents the number of standard deviations a particular value is away from the mean.
2. T-table: The T-table is used to find critical values for tests involving t-distributions. This method is typically used when sample sizes are small or the standard deviation of the population is unknown. Similar to the Z-table, you determine the critical value by finding the t-score corresponding to a given significance level (α) or confidence level. The t-score represents the number of standard errors a particular value is away from the mean.
The choice between using a Z-table or T-table depends on the specific requirements and assumptions of the statistical test being performed. If the sample size is large and the population standard deviation is known, the Z-table is commonly used. On the other hand, if the sample size is small or the population standard deviation is unknown, the T-table is usually employed.
It is important to note that the critical value is determined based on the desired level of significance (α), which represents the probability of making a Type I error (rejecting a true null hypothesis). The critical value ensures that the test has an appropriate level of statistical significance.
Overall, the method used to find the critical value depends on the specific statistical test being conducted and the associated assumptions and requirements of that test.
How does the sample size affect the critical value and the overall statistical analysis?
The sample size can affect the critical value and the overall statistical analysis in several ways:
1. Confidence Level: The critical value is typically used to determine the confidence level of a statistical test. A higher confidence level requires a larger critical value. As the sample size increases, the standard error of the estimate decreases, leading to a decrease in the critical value required to achieve the same confidence level.
2. Margin of Error: The critical value is used to calculate the margin of error in a statistical estimate. The margin of error represents the range within which the true population parameter is likely to fall. With a larger sample size, the margin of error becomes smaller, indicating a more precise estimate, and thus, a smaller critical value.
3. Hypothesis Testing: In hypothesis testing, the critical value is compared to the test statistic to determine if the null hypothesis should be rejected or not. As the sample size increases, the standard error decreases, resulting in a smaller critical value. This means that the test becomes more likely to reject the null hypothesis and detect smaller effects.
4. Power of the Test: The sample size also affects the power of a statistical test, which is the probability of correctly rejecting a false null hypothesis. With a larger sample size, the power of the test increases, allowing for a smaller critical value. This means that the test becomes more sensitive to detecting differences or relationships between variables.
In summary, increasing the sample size generally leads to a decrease in the critical value, indicating a more precise estimation and a higher likelihood of detecting significant effects in statistical analysis.
Are there any common distributions used to find critical values in statistics? If yes, which ones and how are they used?
Yes, there are common distributions used to find critical values in statistics. Some of the most commonly used distributions include the normal distribution, t-distribution, Chi-square distribution, and F-distribution.
1. Normal Distribution: The normal distribution is widely used in statistics, and critical values for this distribution are often expressed in terms of the standard deviation (z-scores). To find the critical value for a given significance level (α) in a normal distribution, you can use a standard normal distribution table or a calculator. The critical value will correspond to the z-score that accumulates α in the tail(s) of the distribution. For example, for a 95% confidence level, the critical value would be 1.96 as it corresponds to the z-score that accumulates 95% in the tail.
2. t-Distribution: The t-distribution is used when the sample size is small or when the population standard deviation is unknown. The critical values for the t-distribution are based on the degrees of freedom (df), which is determined by the sample size. Like the normal distribution, the critical values for the t-distribution can be found using a t-table or a calculator.
3. Chi-Square Distribution: The chi-square distribution is used in statistical tests involving categorical data. The critical values for the chi-square distribution are determined by the degrees of freedom, which depend on the number of categories in the data. The critical values for the chi-square distribution can also be found using a chi-square table or a calculator.
4. F-Distribution: The F-distribution is commonly used in statistics when comparing variances between multiple groups. The critical values for the F-distribution depend on the degrees of freedom associated with the numerator and denominator of the variance ratios being compared. These critical values can be found using an F-table or a calculator.
In summary, different distributions are used to find critical values in statistics depending on the specific scenario and hypothesis being tested. The choice of distribution is based on factors such as sample size, data type, and assumptions of the statistical test being conducted.
Can you provide an example or scenario where finding the critical value in statistics is crucial for accurate data analysis?
Sure! Finding the critical value in statistics is crucial for accurate data analysis in various scenarios. One such example is hypothesis testing.
Let\'s say you are conducting a hypothesis test to determine if there is a significant difference between the mean heights of two populations, population A and population B. Your null hypothesis (H0) states that there is no difference, while the alternative hypothesis (Ha) states that there is a difference.
To perform the hypothesis test, you need to set a significance level (α), which represents the maximum probability of making a Type I error (rejecting H0 when it is true). Commonly used significance levels are 0.05 (5%) and 0.01 (1%).
Once you have determined your significance level, you need to find the critical value associated with it. The critical value serves as a threshold beyond which you can reject the null hypothesis. It is based on the distribution of the test statistic, which depends on the type of test you are conducting.
For example, if you are conducting a two-sample t-test to compare the means of two populations, you can find the critical value by using a t-distribution table or a statistical software. The critical value will be different for different significance levels and degrees of freedom (which depends on the sample sizes and assumptions of the test).
Let\'s say you choose a significance level of 0.05 for your t-test. Looking up the critical value in the t-distribution table, you find it to be 2.048 for a two-tailed test.
After conducting the t-test and calculating the test statistic (t-value), you compare it with the critical value. If the absolute value of the t-value exceeds the critical value of 2.048, you would reject the null hypothesis and conclude that there is a significant difference between the mean heights of the two populations.
By finding the appropriate critical value and comparing it with the test statistic, you ensure that your conclusion about the difference in population means is statistically valid and reliable.
In summary, finding the critical value in statistics is crucial for accurate data analysis, particularly in hypothesis testing scenarios. It helps establish a threshold for decision-making, allowing you to determine whether the results are statistically significant and support the alternative hypothesis.
_HOOK_